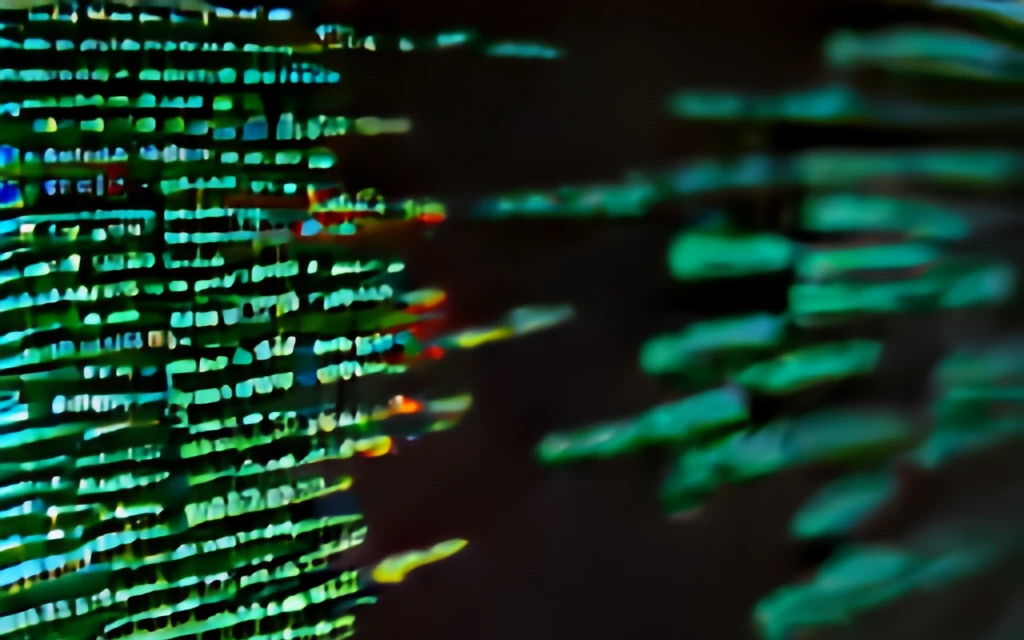
For decades IT architects and developers have dreamed of building fully configurable software that does not require coding. As far back as the 1980s, we heard and dreamt about fifth-generation programming languages and model-based approaches to programming.
The recent arrival of concepts like Composable Business (See bit.ly/3GoJ0O8), which emphasizes the use of modular and configurable blocks to implement business support functions using multiple building blocks only accelerated these trends. Also new practices and patterns like automation, cloud, DevOps, agile development, advanced IDEs (Integrated Development Environments), and last but not least, generative AI (ChatGPT and similar) made coding much faster and more optimal than it used to be just decade ago.
Although the development of no-code or low-code platforms has only accelerated since then, the high-code approach has also been optimized simultaneously. Low-code and no-code still have a way to go to replace the high-code completely. The question remains, though, what to choose, the classical high-code approach, low-code, or no-code approach? Which is better? As usual, the answer is not trivial. It all depends.
Let’s start clarifying what we mean by low-code/no-code and high-code approaches:
- Low-code (no-code) refers to software development platforms allowing users to create applications with minimal or no coding. These platforms often have a visual interface that enables users to build applications by dragging and dropping pre-built components or using predefined templates. Low-code platforms are designed to make it easy for non-technical users or business analysts to create applications without requiring specialized programming knowledge. Examples include Service Now, Microsoft Dynamics 365, Salesforce, Microsoft Power Platform, Appian, and similar solutions.
- On the other hand, high code refers to traditional software development approaches that require extensive coding in a programming language. High code development typically involves writing code from scratch and using libraries and frameworks to build applications. It requires a strong understanding of programming concepts and higher technical expertise. Examples are all major programming languages and development stacks and tools: Python, Java, C#, C++, Intellij, Eclipse, and thousands of associated tools for development and testing.
As we experience each aspect of human activity, development in IT is also a cyclical process. It happens now and then a reevaluation – techniques and practices considered a bleeding edge and modern must give way to procedures and practices once regarded as outdated. The configurable off-the-shelf solutions, either as best-of-suite or best-of-breed solutions, were an obvious no-code/low-code choice just a decade ago. However, a lot has changed around building and implementing IT solutions in the last decade, and the high-code approach has also been improved.
These improvements – such as Agile, CI / CD (continuous integration and continuous deployment), containerization, cloud, IaC (Infrastructure as Code), and DevOps – reduce unit costs of IT systems development and increase fault tolerance. We can now create high-quality software in small increments, often providing measurable business value, and we can withdraw a change with errors at no cost. Modern hyper-shortening business cycles prefer fast point solutions, and all modern IT engineering is suited to such activities – from the cloud, and manufacturing processes, to architecture.
For us architects, this is a world with increasing challenges, but it is often more profitable to make custom software than to implement ready-made “combines.” It is often cheaper to change software than to buy a configurable solution and change the configuration of such a solution.
The no-code/low-code platform economy is absolute. Each configuration option adds an extra dimension of complexity to the solution that must be paid for today, no matter whether we will ever use it. Compared to the tailored solutions created based on actual needs and requirements, there are several economically unnecessary and meaningless functions in a configurable system. Maintaining it also means an additional dimension of complexity in every analysis, test, and each implementation.
Is there, therefore, any point in using no-code/low-code with all these drawbacks?
Low-code and no-code cannot replace coding completely. However, there are still several cases where no-code/low-code platforms are the best fit. That includes:
- Non-technical users or business analysts can use low-code platforms to build applications that automate business processes or improve workflows. This concept is known as citizen development, and it can help organizations quickly respond to changing business needs without relying on IT teams.
- We can use Low-code platforms to build simple applications quickly, which can be helpful in situations where there is a need for rapid application development, such as in startup environments or for small businesses (rapid application development).
- Low-code platforms allow users to quickly build and test prototypes of an application without the need for extensive coding. Such low-code prototyping can help evaluate an idea’s feasibility or gather feedback from stakeholders.
Low-code and no-code are particularly well-suited for implementing standard functionality which is not specific to a particular business domain. Implementing systems and platforms for support functions like sales/CRM (Customer Relation Management), HR (Human Resources), logistics, IT support, and IT infrastructure makes sense. These areas often require a low level of customization. All kinds of enterprise systems like CRM, ERP (Enterprise Resource Planning), ITSM (IT Service Management), integration platforms, and basic infrastructure are often where low-code and no-code can be optimal. The same applies to systems and functions, which are well standardized and used by several actors in the same industry, e.g.:
- OSS (Operation Support Systems)
- BSS (Business Support Systems) solutions
- OT (Operation Technology) systems for energy actors, travel planner systems for mobility actors
- and so on.
On the other hand, the functionality specific to your business and where there are no standard solutions are often targets for tailored high-code implementation. Depending on the complexity and scale of the project, low-code and no-code platforms may not be suitable for large-scale or performance-critical applications, as they may not be able to handle the volume of data or processing requirements. High-code approaches may be ideal for these projects, as they allow for more flexibility and control in the development process.
Low-code and no-code platforms may be suitable for organizations that do not have access to or cannot afford specialized programming resources, as they allow non-technical users to create applications without coding knowledge. On the other hand, high-code approaches may require a more significant investment in training and development resources, as they require a strong understanding of programming concepts.
Low-code and no-code platforms may also offer limited customization options and may need to be able to integrate with other systems or technologies as seamlessly as high-code approaches. This issue may be a significant drawback for organizations that need to integrate their applications with other systems or have specific customization requirements.
Finally, low-code and no-code platforms may struggle to keep up with the rapid technological change and may become outdated or unsupported. High-code approaches may offer more flexibility and adaptability, allowing developers to customize and update their solutions precisely as required.
To summarize. The choice between low-code/no-code and high-code approaches will depend on the specific needs and resources of the organization, as well as the complexity and scale of the project. While low-code and no-code platforms may be suitable for prototyping and testing ideas, creating simple applications quickly, or for non-technical users, they may be less useful for more complex or customized projects that require specialized programming skills. On the other hand, high-code approaches may be ideal for these projects, as they offer more flexibility and control in the development process. However, high-code methods may require a more significant investment in training and development resources. They may not be as suitable for organizations that do not have access to specialized programming resources. There is no single answer to the question of what to choose. As usual, it all depends. However, in any organization and most cases, we should see both low-code/no-code and high-code solutions simultaneously. Many organizations can reduce the use of high-code/tailored solutions to the absolute minimum. We should, of course, attempt to minimize the use of high-code to zero since that is the dream, but that should never be a goal by itself.
Views expressed are my own.